"By 2022, 70% of organizations will rigorously track data quality levels via metrics, increasing data quality by 60% to significantly reduce operational risks and costs."
Source: Gartner, 100 Data and Analytics Predictions Through 2024, Analysts: Graham Peters, Alan D. Duncan, 2020
Organizations that rely on data to drive innovation, maximize revenue opportunities, and make timely decisions, need a scalable and automated way to surface data quality issues across their stack. That’s why at Soda, we placed a lot of importance on our automated Time Series Anomaly Detection capability to make it easy to set up and scalable across the enterprise.
Time Series Anomaly Detection is a new, easy to set up, monitor type available on the Soda Data Observability Platform that can be used on any metrics that unfold over time. Think for example: daily sales, or the percentage of valid values, by year, season, month, or week.
Getting Started with Time Series Anomaly Detection
As part of Soda's Intelligence features, Time Series Anomaly Detection serves data scientists, analysts, as well as platform and data engineers, whose role it is to increase the trust in data and extract its value, so that decisions can be made with confidence.
Time Series Anomaly Detection applies machine learning algorithms to the data quality metrics of each dataset, and learns their behaviour and patterns over time, in order to detect unusual or abnormal data points. The biggest challenge with building a time series anomaly detection system is making sure that that system is able to stand the test of time and to adapt to the data as it changes and evolves.
A common solution or approach for tools built in-house is for data practitioners to set up an alerting system based on thresholds. This can work when there is a good understanding of the underlying data, but it requires expert domain knowledge and constant iterations and adaptations. It also means that the time spent on maintaining an in-house solution, is time that is taken away from modeling and analyzing the data for business insights.
That’s why we built the Time Series Anomaly Detection feature as an out-of-the-box capability. Yes, that’s right - there is no configuration required. No initial thresholds to set up and no complex business logic to codify.
Soda builds a predictive model that uses historical data to understand the common trends and seasonal patterns of the time series data. Each measurement is a point over time. When measurements are anomalous, they will be flagged with a colored dot, denoting whether it is in the ‘warning’ (yellow dot) or ‘critical’ (red dot) zones, based on the severity of the anomaly.
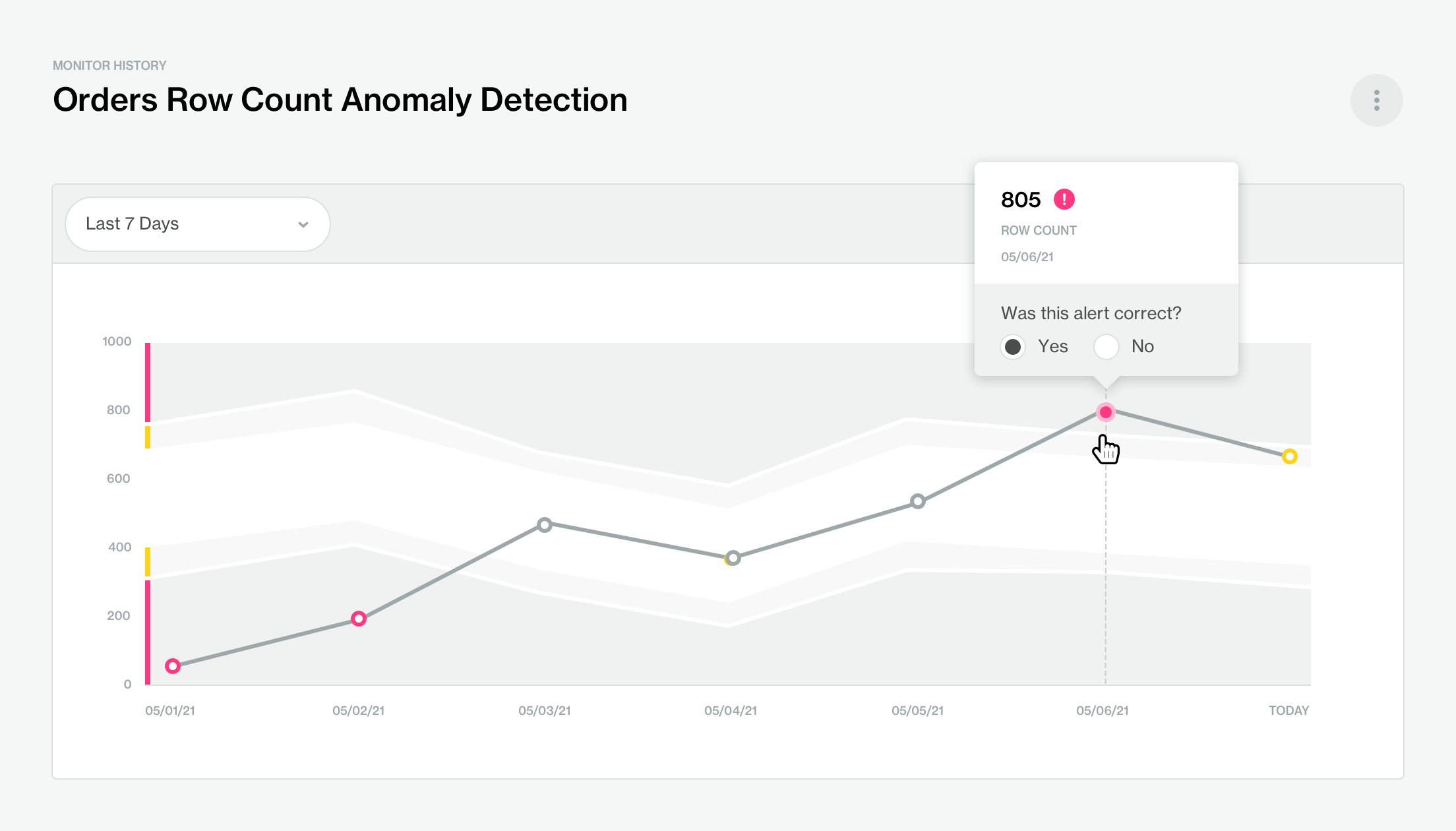
Remove Gaps in Observability Across Data
Automation becomes better when an algorithm can be trained and when it continues to learn and adapt to user feedback. For this to happen, it is sometimes necessary for the data subject matter experts or the data stewards to adjust and fine-tune it. And that’s another advantage of using Time Series Anomaly Detection by Soda as our algorithm can learn from user feedback.
If a user notices that a data point is flagged as anomalous while it in fact is an expected variation, such as a drop in the number of orders over the weekend for an office supplies online retailer, the user can un-flag this anomaly via annotations and the model will change its assumptions the next time it runs.
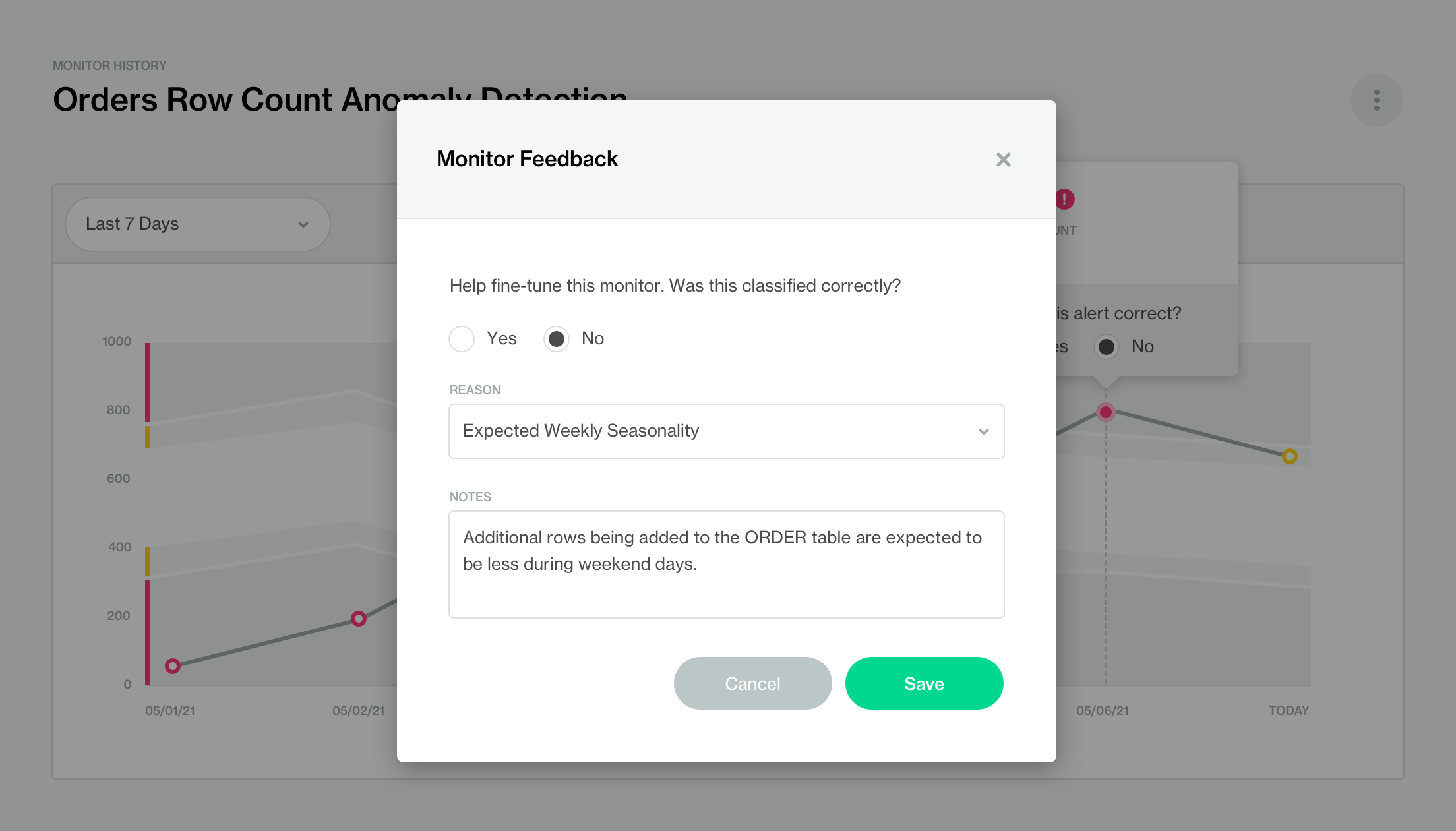
Sometimes even machines need a helping hand - especially if the data is idiosyncratic to your business. Another benefit of allowing user feedback to tweak the internals of the algorithm is that it will reduce the noise or “alert fatigue” faster and allow you to focus on the things that really matter.
As a side note, in addition to Time Series Anomaly Detection, users also have the capability to manually set up changes over time or threshold-based monitors in Soda. This is an alternative, if you have knowledge of how a dataset might behave that you can define and set the levels for what good data looks like and what anomalous behaviour is.
Because we believe that data quality is a team sport, Soda’s platform is built to enable collaboration. Alerts are routed to the right people, defined by roles and responsibilities, for triage and investigation as soon as they occur. Everyone who has a stake in the data can understand it, trust it, and stay on top of it. Users can focus their attention where their skills have an impact on the business.
Unknown Causes Defined by Their Upstream & Downstream Impact
The rippling effects and the cost of bad data are recognized by organizations, both large and small. Bad data quality is costly not only in terms of time and resources but also in its impact on the opportunities to drive innovation, make timely decisions, maximize revenue opportunities, and ultimately, reputation.
Soda detects the anomalous or outlier data and bubbles it up to the surface to enable the right people in the team to analyze and discover the root cause of the incident. But, it doesn’t, and shouldn’t, stop here.
The definitive root cause of a bad data incident is often unknown, but the incident will often be defined by its impact on the business.
Root Cause Analysis to Prevent Future Issues
Understanding and getting to the root cause of anomalous data, as well as being able to proactively prevent future data issues, requires data teams to investigate deeper and wider into the data, beyond just the anomalous data. The hard-to-find-errors and their root causes are often buried deep within the data and we know that it is almost never one single issue, but it is in fact several missteps.
The data team needs to be able to focus on fixing the root cause rather than applying short-term fixes downstream.
The 3 recommended actions that we advise data teams to undertake for root cause analysis are:
- Analyze commonalities or differences in failing and passing rows
- Use data lineage to trace errors right back to the root cause and understand the impact at the highest upstream point and on the dependencies downstream
- Examine analytics code changes and identify the impact of new code releases

Control and Trust for Everyone
Tackling the root cause analysis head-on gets the data to a stable future-proof state, helps data teams prevent recurring issues and stabilizes the data quality across the organization. That's what we think incident resolution and getting ahead of quality issues should look like and why we wanted Time Series Anomaly Detection to be so easily accessible. It can be used across a number of industries and for a variety of purposes.
Whilst there are many types of anomalies, the anomalies that are important for businesses are additive outliers (the unexpected spikes or the sudden drops in the data); temporal changes (trends); and seasonal level shifts. You’ll see examples of these in e-commerce: sudden changes in the number of completed transactions or unexpected surges in demand; hard-to-find errors such as a mispriced item or an inaccurate tax calculation. In manufacturing: tracking and monitoring equipment and machinery with connected IoT devices to prevent downtime and disruptions. And in finance: tracking and monitoring the price of a collection of assets over time to detect potential surges or erroneous data aggregations.
Data Enables Transformation and Change
As many businesses shift their models to focus on growing their digital product portfolio and creating that ultimate online experience, the need for good quality data increases.
Zalando SE is an online European fashion retail platform, founded in 2008 in Berlin, Germany. Good quality data and technology are an important part of the culture with teams building data products on which all business decisions are based. It’s ambition is to be the starting point for fashion across Europe. With 42 million customers in 20 markets, it is one of the businesses that benefited from the pandemic as shared by Dr. Alex Borek during Soda Live. Alex explained that Zalando was “supporting offline retailers to get online, as a way to give back to the community, as a lot of retailers and fashion companies struggled as physical shops closed.”
Businesses that can use data confidently, are also better prepared to understand the sudden and ever-changing needs of their customers and better anticipate the increase in e-commerce transactions and the rise in online activity to align on priorities and deliver a superb digital customer experience.
NN Investment Partners (NN IP) is a Dutch asset manager with headquarters in The Hague, The Netherlands, and offices in 15 countries globally. At NN IP, data and technology is used for investment decisions - fundamental analysis, real time data, and artificial intelligence help NN IP invest responsibly and get the maximum returns for their customers. “The entire customer experience at NN Investment Partners is actually secured through proper checks and balances around data,” Martijn Spaan, Head of Data & Oversights shared during Soda Live. Combining human creativity and machine rigour leads to stronger decision-making.
The Benefits of Observing Anomalous Behavior in Data
Time Series Anomaly Detection in Soda is always on. As soon as datasets are onboarded, anomaly detection is automatically set up for the key data quality metrics, such as row count (volumes) and arrival times (freshness).
Many of us at Soda, at one time, have been part of a data team working late into the night to identify and resolve data issues. We understand the fear of not knowing, finding out about data issues too late, and the pain of putting things right after the fact. So much time is spent - and wasted - on firefighting issues.
The goal is to enable everyone with data. The value is in the insights that the anomalies provide for users to intervene, initiate further analysis, and take action. For any organization, this will result in control and trust in the data, and business benefits including increased revenues and efficiencies, improved customer experience, decreased risk, and competitive advantage.
All organizations should be able to transform and automate with data that they can trust.
If you would like to put Soda’s Time Series Anomaly Detection to work on your data, register for a free trial. If you need sample data, search Google’s dataset.
What’s Coming Next
At Soda, this is just the start of our journey to build intelligent features that give data teams the capability and control to create trusted, quality data for an organization to drive automated processes, efficiencies, and insights and decisions.
Here is a preview into what we are solving next:
- Automatic monitor suggestions based on patterns and behaviour in your data
- Automatically grouping alerts that stem from similar data flows
- Helping you get to the root cause of data issues by analysing diagnostics data
To stay up to date, join our Slack Community or sign up to stay connected with Soda news and updates.
To request a demo, please contact us.