Data contracts are gaining traction because they bring about encapsulation in data engineering. Encapsulation in software engineering is a principle that intends to keep different parts of a system separate so that changes in one portion does not disrupt the rest of the system unintentionally. In data engineering however, this principle has been ignored.
Traditionally, data management has focused on detecting and fixing issues after they occur. Data contracts shift this approach by preventing issues at the source. They create well-defined agreements between data producers (who generate and manage data) and data consumers (who rely on it).
These rules work like APIs in software, ensuring that the data follows a fixed format and quality standard. This makes it easier to trust and use the data without worrying about sudden errors.
Tutorial: Implementing Data Contracts at Scale
In this blog, I'll guide you through writing and verifying data contracts while emphasising on the best practices. We will also see how multiple contracts can interact with each other seamlessly within a simple supply chain database. This is an end to end blog that helps you start a data contract from scratch to scaling it.
Setting up Soda for Data Contracts
For this tutorial, we’ll use Soda. If you already have it installed and configured to a data source, you can skip this section and scroll down to Writing Your First Data Contract
Step 1 : Check Prerequisites
Soda requires a Python version above 3.8. I am using Python 3.13 along with pip version 24.2. I have already set up Docker Desktop and PostgresDB. There are ways to setup Soda without Docker. To explore other options please refer to the documentation.
Step 2 : Install Soda for PostgreSQL
Open your terminal and create a directory for your Soda project:
mkdir dc_from_scratch
cd dc_from_scratch
It’s best practice to install Soda in a virtual environment to keep dependencies clean. Run:
python3 -m venv .venv
source .venv/bin/activate # Activates the virtual environment
Since I’m using a PostgreSQL database to store my data, I’ll install soda-postgres.
If your data lives elsewhere, install the appropriate Soda connector. Inside your virtual environment, run the following command:
pip install -i <https://pypi.cloud.soda.io> soda-postgres
Verify the installation and you’re good to go.
soda -help
Step 3 : Set Up a Sample PostgreSQL Database Using Docker
To enable you to take a first sip of Soda, you can use Docker to quickly build an example PostgreSQL data source against which you can run scans for data quality. The example data source contains data for AdventureWorks, an imaginary online e-commerce organization.
- Open a new tab in Terminal.
- If it is not already running, start Docker Desktop.
- Run the following command in Terminal to set up the prepared example data source.
docker run \\
--name sip-of-soda \\
-p 5432:5432 \\
-e POSTGRES_PASSWORD=secret \\
sodadata/soda-adventureworks
Wait for the message: "Data system is ready to accept connections."
This means the database is up and running! We can move on to writing a contract.
Writing Your First Data Contract
A data contract is a formal agreement between data producers and consumers that defines the structure, quality, and expectations of data.
Soda data contracts is a Python library that enforces data quality by verifying checks on newly produced or transformed data.
Contracts are defined in YAML files and executed programmatically via the Python API, ensuring that data meets predefined standards before it moves downstream.
While still experimental, data contracts can be integrated into CI/CD workflows or data pipelines to catch issues early. Best practice involves verifying data in temporary tables before appending it to larger datasets.
pip install soda-core-contracts -U
I have already created a database called "dairy_supply_chain" in my local Postgres dbms. The user and password attributes are not related to Soda but related to the database. Soda API will use it to access the database.
Next, in your project’s root directory (dc_from_scratch/
), create a data source configuration file named data_source.yml
.
The data source file is a configuration file that defines how Soda Core connects to a specific data source .
name: local_postgres_
type: postgres
connection:
host: localhost
port: 5432
database: dairy_supply_chain
user: sample_user
password: secret
Create a directory to store your data contracts, and inside it, create a separate .yml
file for each dataset. To keep things simple, I name each contract file after the dataset it corresponds to.
For example, here’s the Factories.contract.yml
file for the Factories
dataset.
data_source: local_postgres
dataset: Factories
columns:
- name: factory_id
data_type: integer
- name: name
data_type: text
checks:
- type: no_invalid_values
valid_min_length: 2
- name: location
data_type: text
- name: contact_info
data_type: text
optional: true
checks:
- type: no_duplicate_values columns: ['contact_info' ,]
Each contract defines the required columns, their data types, and validation checks as necessary. Every column in the dataset must be explicitly listed with its data type.
.png)
Verifying a Data Contract
Next, we need to create a main.py
file that will be the one responsible for executing data contract verification using Soda Core. If any rule is violated, the script identifies the failure and provides details for further action.
It first loads the data source configuration from data_source.yml
, which contains database connection details. Then, it runs a contract verification process by loading the contract file (Factories.contract.yml
) and checking if the dataset meets the defined rules.
If the verification passes, it prints a success message; otherwise, it prints a failure message along with details of what went wrong.
import os
import logging
from soda.contracts.contract_verification
import ContractVerification, ContractVerificationResult
data_source_file = "data_source.yml"
print(f"Running contract verification")
contract_verification_result: ContractVerificationResult = (
ContractVerification.builder()
.with_contract_yaml_file(".../Factories.contract.yml")
.with_data_source_yaml_file(data_source_file)
.execute()
)
if not contract_verification_result.is_ok():
all_passed = False
print(f"Verification failed")
print(str(contract_verification_result))
else:
print(f"Contract verification passed.")
Run this script to verify the contract.
You might run into a ModuleNotFoundError: No module named 'soda data_sources spark_df_contract_data_source’
To resolve this: pip3 install soda-core-spark-df
Before we jump into developing the rest of the contracts, let's see some errors you might run during the process and how to deal with them.
Enforcing Data Contracts Across Multiple Tables
Let’s step back and look at the bigger picture how the entire database fits together.
This is the sample dairy supply chain database. It is designed to track the flow of dairy products from factories to warehouses, then to retailers, ensuring smooth supply chain operations.
- Factories are at the core, where products are manufactured.
- Warehouses act as distribution points, storing products before they reach retailers.
- Retailers are the final stop, selling products to customers.
- Inventory and stock tables at the levels help manage quantities and track updates.
Each dataset has a clear role and depends on others in different ways. Your contracts should reflect these differences and enforce rules that maintain data integrity.
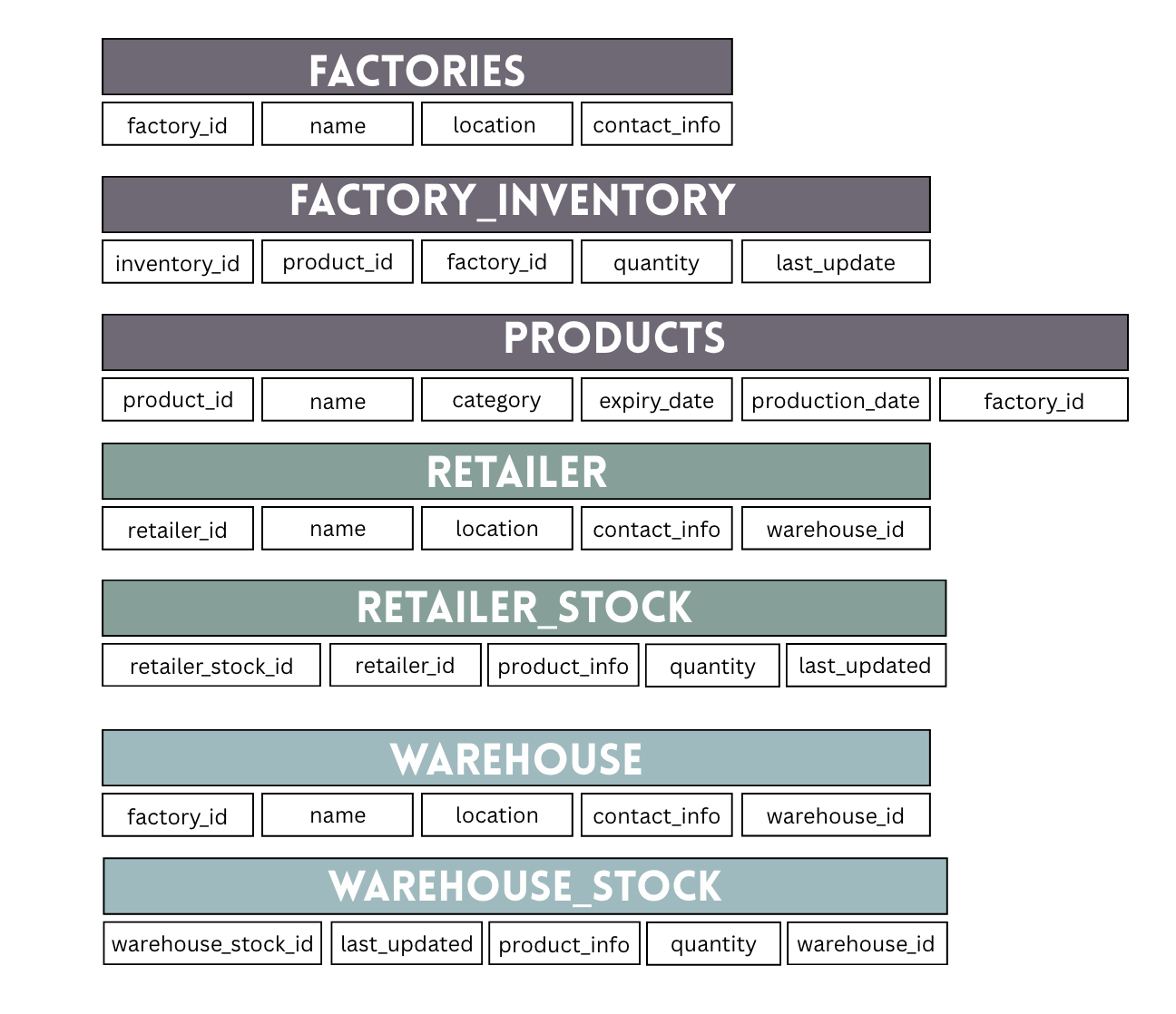
I have written down contracts for other datasets similar to the factories dataset. I discuss some unique checks that you can use in the contracts later in the blog. For now, your directory structure will be similar to this:
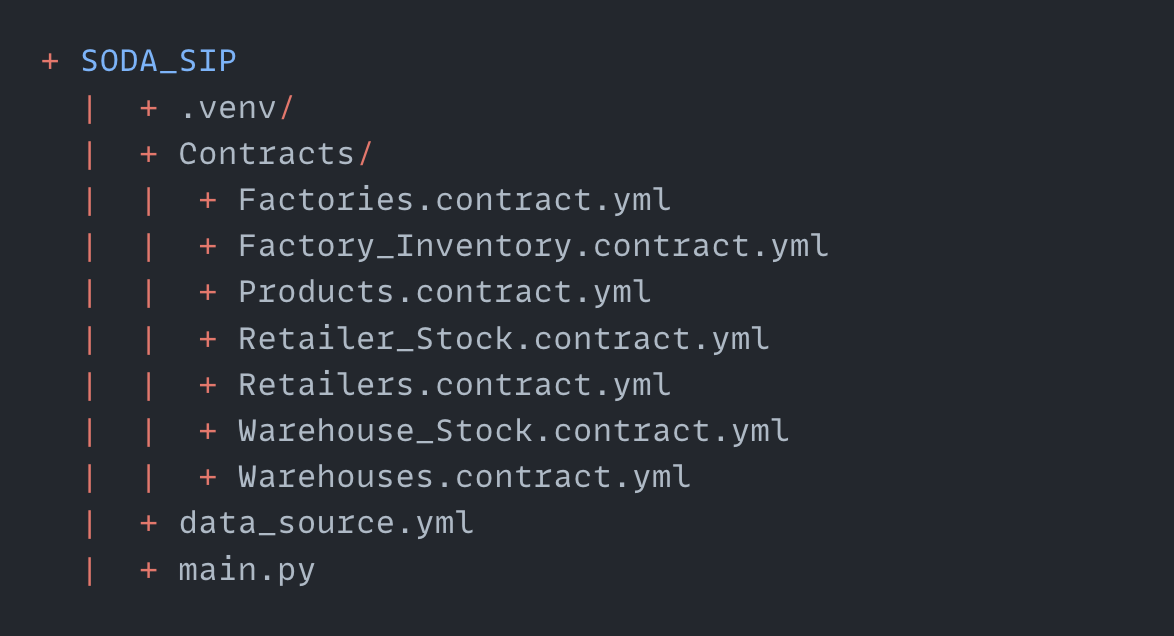
Dynamic Data Contracts
Our script processes each contract one by one in a loop, verifying them sequentially. While this approach works for a controlled setup, it doesn't reflect how data flows in the real world. There is no dynamic data ingestion, we are simply running through static contracts after the data has already been added.
If you noticed so far execution errors cause the process to exit but the pipeline continues to be executed even when there are check failures. This would definitely defeat the point of contracts.
Instead of verifying all contracts in a fixed sequence, we need to switch to a more event-driven approach, where checks are triggered dynamically based on incoming data. In this section, we will be scaling the complexity of the data pipeline now that we have readied our contracts.
Before everything, connect the database with your Python environment. Like so:
import psycopg2
from soda.contracts.contract_verification
import ContractVerification, ContractVerificationResult
DB_PARAMS = {
"dbname": "dairy_supply_chain",
"user": "",
"password": "",
"host": "",
"port": ""
}
def connect_db():
try:
conn = psycopg2.connect(**DB_PARAMS)
return conn
except Exception as e:
print(f"❌ Error connecting to database: {e}")
return None
The key principle here is that a contract should only be verified when its dependencies are satisfied.
A well-implemented data contract functions as a gatekeeper for updates. Instead of blindly allowing changes, it verifies and permits only high-quality data to enter the system.
For instance, the Products
dataset acts as a foundation for inventory tracking.
If a new product is added or updated, it must meet all data quality standards before updating Inventory_Stock. This stops incorrect records from affecting historical data and keeps information accurate for all stakeholders.
Also not all datasets are dependent on one another. If a contract fails for a specific dataset, unrelated datasets should not be affected. For example, even if Products
verification fails, the Warehouses
dataset can still process updates, since it operates independently.
Of course, a real-world implementation of this system would be more sophisticated, often involving database triggers and queuing mechanisms to manage contract execution efficiently.
However, given the scope of this blog, we’ll focus on a simplified version to illustrate the concept. This will help us understand the core principles of implementing data contracts at scale.
Add a Dependency_Map that tracks the relation of each dataset and the corresponding contracts, data owner and related datasets.
DEPENDENCY_MAP = {
"Products": (
"Contracts/Products.contract.yml",
"Factory Production Head - Mark Johnson",
[]
),
...rest of the datasets
}
When a query is executed, the script first checks the dataset’s dependencies using DEPENDENCY_MAP
. Each dataset has a corresponding contract and data owner. Before an update, it verifies that all required contracts have passed. If a dependency fails, the update is blocked, and an alert is printed.

If all dependencies are satisfied, the script connects to the PostgreSQL database and executes the query. Any errors encountered during insertion are caught and displayed, preventing system crashes.
def run_query(query, dataset):
contract_file, data_owner, dependencies = DEPENDENCY_MAP[dataset]
for dependency in dependencies:
dep_contract, dep_owner, _ = DEPENDENCY_MAP[dependency]
if not verify_contract(dep_contract, dep_owner):
print(f"⛔ {dataset} update blocked due to {dependency} contract failure.")
return
conn = connect_db()
if conn:
try:
with conn.cursor() as cursor:
cursor.execute(query)
conn.commit()
print(f"Data inserted into {dataset}")
except Exception as e:
print(f"Error inserting into {dataset}: {e}")
finally:
conn.close()
if __name__ == "__main__":
print("...................")
run_query(
"INSERT INTO Products (product_id, name, category,
expiry_date, production_date, factory_id)"
"VALUES (7, 'Milk', 'Dairy', '2025-01-01',
'2025-03-01', 1);","Products"
)
print("...................")
run_query(
"INSERT INTO Factory_Inventory (inventory_id, product_id,
factory_id, quantity, last_updated) "
"VALUES (3, 5, 1, NOW());",
"Factory_Inventory"
)
print("...................")
run_query(
"INSERT INTO Warehouses (warehouse_id, name, location,
contact_info, factory_id)"
"VALUES (6, 'Warehouse F','City G', +197883205 , 1);",
"Warehouses"
)
print("\nPipeline Execution Done.")
The output of the above script is as follows:
.png)
On inserting data rows, a contract check for Products detected an issue: "Production date must be before expiry date."
The contract expected zero violations (invalid_date_order_count = 0), but instead, two violations were found. This failure triggered a notification to the Factory Production Head, Mark Johnson, since they are the data owner.
Because Factory_Inventory
depends on Products
, and the Products
contract failed, the system prevented any updates to Factory_Inventory
.
A message explicitly states: "Factory_Inventory update blocked due to Products contract failure."
Since Warehouses
is independent of Products
, its update proceeded without any issues.
Who is Responsible for Data Contracts?
Who is responsible when things go wrong during contract verification?
Think of a data pipeline like a railway system. Data engineers are the track builders and maintenance crew i.e. they lay the tracks, build operations, and keep everything running efficiently. But when a train (data) derails due to incorrect cargo (bad data), do we call the track builders? No. We contact the cargo supervisor who the person responsible for loading the material.
That’s who the data owner is. Basically:
- Data Engineers set up pipelines, verify proper data ingestion, and maintain the infrastructure.
- Data Owners are responsible for the correctness of the data itself. They are usually domain experts, or someone who supervises the data collection process for the corresponding dataset.
Here, in our supply chain, a Factory Manager might be the data owner for factory inventory, while a Retail Supervisor owns retailer stock data.
Creating a data contract is the first step in establishing ownership. Datasets without a designated owner are inherently unstable, often leading to failures in downstream consumer assets. As a data consumer, you should prioritise using datasets with clear ownership i.e. an accountable individual you can reach out to for issues or clarifications.
.png)
Take a look at the two alerts in the image above.
The second alert is much more useful because it:
- ✅ Shows what failed
- ✅ Confirms it’s a data issue, not an execution error
- ✅ Identifies who should take action
Such alerts are called “Contextual Alerts” and they help the system become transparent and make the issue resolution process much faster.
We already implemented some of the logic to make contextual alerts by differentiating between check fail and execution error.
Now, we simply need to just add data owners into our contract verification logic:
if contract_verification_result.has_failures():
print(f"Failed checks detected in {contract_file}:")
print("-" * 80)
print(str(contract_verification_result))
print("-" * 80)
print(f"🔔 Notify: {data_owner} should review this issue.")
.png)
See how perfect!
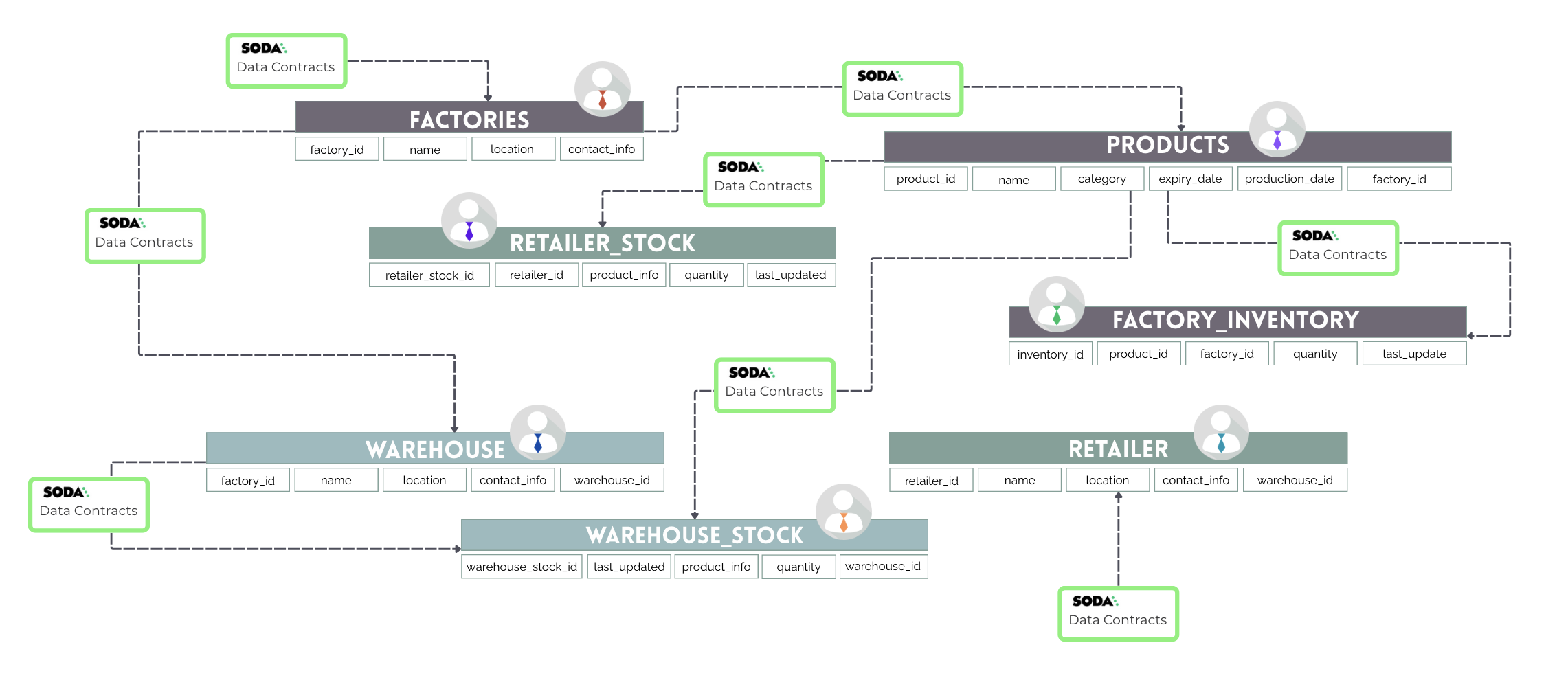
Writing contracts should be after a discussion with team to make sure the expectations are well aligned. Always keep the schema of the dataset with you while writing the contract.
Important Data Contract Checks
Now that we understand the basic flow of contract verification and have setup a simple scenario with the best practises, we can solely focus on increasing the complexity of the checks within the contracts.
Check 1: Quantity Is Never Negative
This check guarantees that the quantity column only contains values greater than or equal to 0, preventing negative numbers from entering the system.
- name: quantity
data_type: integer
checks:
- type: no_invalid_values # Ensures certain values are not allowed
id: positive_quantity_check # Unique identifier for the check
valid_min: 0 # Sets the minimum acceptable value as 0 (no negatives)
Check 2: Category Is Always a Valid Value
The category
field must always have valid values because incorrect or unexpected entries can cause data inconsistencies, reporting errors, and system failures when filtering or aggregating data. A similar check to this would be to standardise unit values are only "kg", "liters", or "pieces".
- name: category
data_type: text
checks:
- type: no_invalid_values
id: category_values_check
valid_values: ["Dairy", "Bakery", "Beverages", "Snacks", "Frozen", "Produce"]
Check 3: Expiry Date and Production Date Must Be Valid
Another column of interest here is the expiry_date column and production_date.
If a product expires before or on the day it was produced, it’s clearly an error. This check prevents bad data that could lead to incorrect inventory decisions, faulty reporting, and misleading shelf-life calculations. It brings logical consistency in product timelines.
checks:
- type: metric_expression
metric: invalid_date_order_count
expression_sql: |
COUNT(CASE WHEN production_date >= expiry_date AND
production_date IS NOT NULL AND expiry_date IS NOT NULL THEN 1 END)
must_be: 0
name: Production date must be before expiry date
The check below verifies that all production dates are either today or in the past, maintaining data accuracy.
- type: metric_expression
metric: future_production_date_count
expression_sql: |
COUNT(CASE WHEN production_date > CURRENT_DATE AND
production_date IS NOT NULL THEN 1 END)
must_be: 0
name: Production date must not be in the future
I have purposefully added a wrong value in the table, let's run the contract again,
.png)
Check 4 : Filter SQL
This check enforces a minimum value of 120 in the quantity column, but only for specific retailers (retailer_id IN (1, 2)).
It is useful where different business rules apply to different entities.
Some other scenarios where such logic would be applied:
- Minimum account balance: Maintain a balance above a set threshold for premium customers.
- Minimum age restriction: Require a minimum age of 18 for accounts that need legal adult status.
- Salary validation: Enforce a minimum salary for specific job roles.
- name: quantity
data_type: integer
checks:
- type: no_invalid_values
id: temp
valid_min: 120
filter_sql: retailer_id IN (1, 2)
.png)
If you need to learn about checks that were not covered in this blog then please explore this documentation. It details all the checks and related how-tos for your Soda data contracts.
Conclusion
Data contracts are a fundamental shift in how we manage and enforce data quality. They move us from a reactive approach to a proactive agreement between data producers and consumers. In this blog, we covered the complete journey of implementing data contracts, from understanding their role to setting up the necessary tools and ensuring best practices.
We started by defining what data contracts are and why they are important for building reliable data pipelines. Then, we covered the technical setup, including how to configure Soda and PostgreSQL, along with the Python scripts needed to integrate contracts into a real-world data environment.
After setting up the tools, we wrote and verified our first contract, ensuring it met the required data quality standards. We also explored best practices, focusing on data ownership, contextual alerts, and structured validation checks to prevent unexpected failures.
Finally, we looked at how to scale data contracts in a supply chain database. This allowed multiple contracts to interact without causing downstream disruptions.
Appendix: Debugging and Error Handling
When verifying your contracts, you'll encounter two types of errors: Failed Checks and Execution Errors.
The table below compares the two:
To simplify debugging, you can use either of the two methods below to display errors and results.
Method 1 : Test for the result and get a report.
# Method 1: Using is_ok() to check the result
if not contract_verification_result.is_ok():
all_passed = False
print(f"Verification failed for {contract_file}:")
print("-" * 80)
print(str(contract_verification_result)) # Get the full report
print("-" * 80)
else:
print(f"Contract verification passed for {contract_file}. ")
Method 2: Append .assert_ok() at the end of the contract verification result which produces a SodaException when a check fails or when execution errors occur. The exception message includes a full report.
# Method 2: Using assert_ok() will raise an exception and
stop execution on failure
try:
contract_verification_result.assert_ok()
print(f"Contract verification passed for {contract_file}.")
except Exception as e:
print(f"Verification failed for {contract_file}:")
print("-" * 80)
print(str(e)) # Exception message includes the report
print("-" * 80
print("Stopping execution due to critical failure.")
exit(1)